Quality Image Annotation: A Catalyst for Business Growth in Software Development
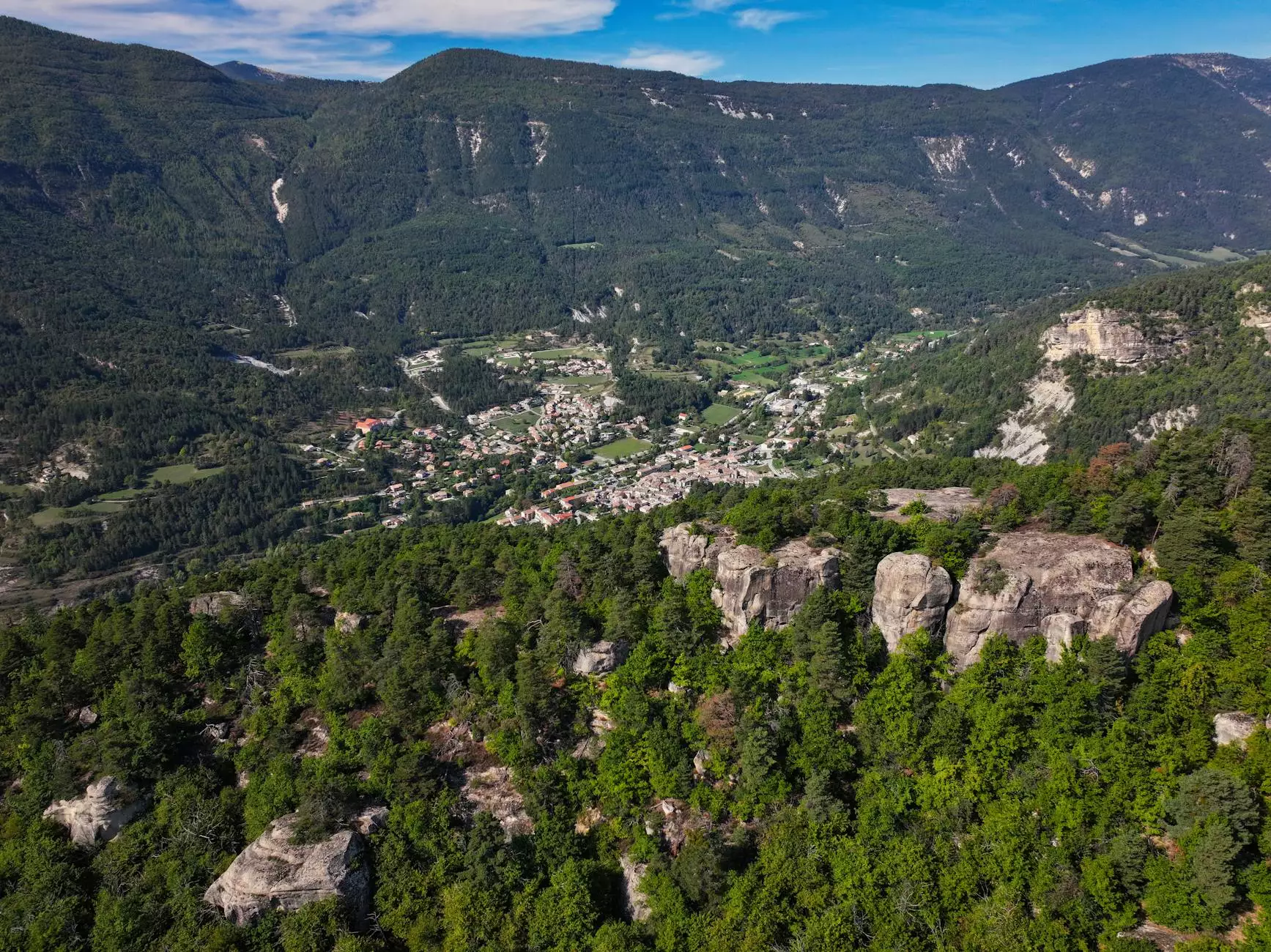
In the ever-evolving landscape of software development, the need for precise and efficient processes is paramount. One of the critical components that can accelerate this process is quality image annotation. This article explores the value of quality image annotation, particularly in its application within software development, and how it serves as a vital tool for businesses striving for excellence.
Understanding Quality Image Annotation
Quality image annotation refers to the process of labeling images with metadata, which can include text descriptions, bounding boxes, or semantic segmentation. The aim is to provide a clear understanding of the content within the images. This meticulous process is essential for training machine learning models, which require high-quality annotated data to achieve superior performance levels.
The Importance of Quality in Image Annotation
While image annotation can be a straightforward task, achieving quality image annotation requires expertise, attention to detail, and a streamlined workflow. High-quality annotations enable businesses to:
- Enhance Model Accuracy: Accurate labels lead to more efficient learning by algorithms, which in turn improves the performance of AI and machine learning models.
- Reduce Errors: Quality annotation mitigates the risks of misclassification, which can significantly hamper business operations and decision-making.
- Save Time and Costs: Investment in quality annotation upfront pays off by reducing the time needed for model retraining and improving overall project timelines.
The Role of Quality Image Annotation in Software Development
In the context of software development, image annotation plays a pivotal role in various applications, particularly in fields such as computer vision, healthcare, autonomous vehicles, and robotics. Here’s how:
1. Enhancing Computer Vision Applications
Computer vision technology relies heavily on accurately annotated images to train models that can interpret visual data. For instance, applications like facial recognition or object detection require extensive datasets annotated with details that help the software learn from them. By utilizing quality image annotation, companies can ensure their models recognize and interpret images effectively, leading to improved functionality.
2. Advancing Healthcare Solutions
In the healthcare industry, quality image annotation is essential for developing diagnostic tools and systems that analyze medical imagery, such as MRIs, CT scans, or X-rays. Properly annotated images enable better training of diagnostic models, which can lead to enhanced patient outcomes through quicker and more accurate diagnoses.
3. Enabling Autonomous Vehicles
Autonomous vehicles rely on a range of sensors and cameras to understand their surroundings. Quality image annotation helps in labeling objects in the vehicle’s environment, which is crucial for safe navigation. Annotations provide the necessary information for training models that recognize obstacles, pedestrians, and road signs.
4. Revolutionizing Robotics
Robotics, much like computer vision, benefits significantly from quality image annotation. Robots need to understand their environment to interact with it effectively. Annotations can guide robots on how to manipulate objects or perform tasks, ensuring precision in operations across various settings, from manufacturing to personal assistance.
Quality Image Annotation Techniques
To achieve exemplary results in image annotation, various techniques and methodologies can be employed. Here are some notable ones:
1. Manual Annotation
Despite being labor-intensive, manual annotation performed by skilled annotators tends to yield the most accurate results. Human annotators can apply contextual knowledge to ensure that annotations are not just correct but also nuanced.
2. Automated Annotation
With advancements in AI and machine learning, automated annotation tools are becoming increasingly popular. These tools use algorithms to apply annotations quickly and at scale. However, while they are efficient, the quality may vary, necessitating manual checks for accuracy.
3. Semi-Automated Annotation
This hybrid approach combines the strengths of manual and automated methods. It allows machines to perform initial annotations, which are then refined by human annotators, ensuring balance between efficiency and quality.
Challenges in Achieving Quality Image Annotation
While the importance of quality image annotation is evident, achieving it can be laden with challenges which include:
- Diversity of Data: Images can vary widely in terms of content, quality, and context. Ensuring consistent quality across diverse datasets can be difficult.
- Cost Constraints: High-quality annotation often requires significant investment in resources and personnel, which may be a barrier for some businesses.
- Scalability: As projects grow, maintaining high-quality standards while scaling up annotation efforts can present logistical challenges.
Best Practices for Quality Image Annotation
To overcome challenges and ensure effective quality image annotation, businesses should consider the following best practices:
1. Define Annotation Guidelines
Establish clear and concise guidelines for annotators. These should outline labeling protocols, examples of common cases, and any specific nuances that are essential for the project.
2. Invest in Training
Ensure that annotators are well-trained. This includes providing training on the tools used, understanding project-specific requirements, and being aware of potential biases.
3. Conduct Regular Quality Assurance
Implement a quality assurance process that includes regular audits of annotated data. This allows businesses to identify errors early and rectify them before they impact the project.
4. Leverage Technology
Utilize tools that facilitate collaboration among annotators and improve efficiency. Slashing time spent on repetitive tasks can yield significant overall efficiency without sacrificing quality.
Conclusion: Quality Image Annotation as a Competitive Advantage
In conclusion, quality image annotation is not just a technical necessity; it is a competitive advantage that can propel businesses in the software development sector to new heights. By investing in quality annotation processes, businesses can enhance the accuracy of their AI models, accelerate project timelines, and improve overall outcomes. As technology continues to advance, embracing quality image annotation will be essential for companies looking to thrive in a data-driven world.
At keymakr.com, we specialize in providing high-quality image annotation services designed to meet the specific needs of your software development projects. Our commitment to quality ensures that your models will perform at their best, ultimately driving business success and innovation.